دانلود رایگان مقاله چارچوبی برای تشخیص تصادفی و طبقه بندی سناریوهای اینترنت اشیای چندگانه – سال 2021
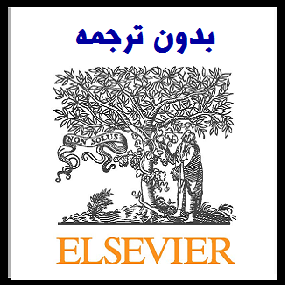
مشخصات مقاله:
عنوان فارسی مقاله:
چارچوبی برای تشخیص تصادفی و طبقه بندی سناریوهای اینترنت اشیای چندگانه
عنوان انگلیسی مقاله:
A framework for anomaly detection and classification in Multiple IoT scenarios
کلمات کلیدی مقاله:
تشخیص ناهنجاری، اینترنت اشیا، اینترنت اشیاple چندگانه، MIoT، بررسی ناهنجاری، مشکل رو به جلو، مشکل معکوس
مناسب برای رشته های دانشگاهی زیر:
فناوری اطلاعات، کامپیوتر
مناسب برای گرایش های دانشگاهی زیر:
شبکه های کامپیوتری، اینترنت و شبکه های گسترده
وضعیت مقاله انگلیسی و ترجمه:
مقاله انگلیسی را میتوانید به صورت رایگان با فرمت PDF با کلیک بر روی دکمه آبی، دانلود نمایید. برای ثبت سفارش ترجمه نیز روی دکلمه قرمز رنگ کلیک نمایید. سفارش ترجمه نیازمند زمان بوده و ترجمه این مقاله آماده نمیباشد و پس از اتمام ترجمه، فایل ورد تایپ شده قابل دانلود خواهد بود.
فهرست مطالب:
Abstract
Keywords
1. Introduction
2. Related work
Surveys and taxonomies.
Anomalies in generic networks.
Anomalies in social networks.
Other approaches.
Classification of our approach.
3. The MIoT paradigm
4. Modeling anomalies in an MIoT
4.1. Definition of anomaly taxonomies
4.1.1. Presence anomalies vs success anomalies
4.1.2. Hard anomalies vs soft anomalies
4.1.3. Contact anomalies and content anomalies
4.2. Formalization of anomalies
4.2.1. Presence-Hard-Contact anomalies
4.2.2. Success-Hard-Contact anomalies
4.2.3. Presence-Soft-Contact anomalies
4.2.4. Presence-Hard-Content anomalies
5. Investigating the origins and effects of anomalies in an MIoT
5.1. Forward problem
5.2. Inverse problem
6. Use case and experiments
6.1. A smart city use case
6.2. Experiments
6.2.1. Description of the testbed
6.2.2. Analysis of the forward problem
6.2.3. Analysis of the inverse problem
7. Conclusion
CRediT authorship contribution statement
Declaration of Competing Interest
Acknowledgments
References
قسمتی از مقاله انگلیسی:
1. Introduction
In the Concise Oxford Dictionary,1 anomaly is defined as ‘‘something that deviates from what is standard, normal, or expected’’. If regularities allow investigating the general characteristics of a complex system, anomalies allow the uncover and analysis of unexpected features that might not be otherwise discovered. For this reason, the detection of anomalies has become very important in data analytics, and is widely investigated both in statistics and machine learning [1–3]. The relevance of anomaly detection is universally acknowledged, since data anomalies are at basis of significant events and patterns. Example application domains include: privacy and cybersecurity [4,5]; fault detection [6]; ecological disturbances [7]; communication networks [8]; social media life [9–12]; and gene regulation [13, 14]. In recent years, anomalies have been widely investigated in social networks to detect fraudulent individuals [15,16], spammers [17,18], malicious behavior, and so forth. Even more recently, anomaly detection has been analyzed in contexts where more social networks interact with each other [19], thus going from social networking into social internetworking. Social internetworking is certainly one of the frontiers of social network analysis, since people tend to have multiple social network accounts and can, thus, become ‘‘social bridges’’. Furthermore, all sorts of networked objects are getting increasingly smart and social, giving rise to the so-called Smart Objects (SOs) and revolutionizing both the Internet of Things (IoT) and the Social Internet of Things (SIoT) [20]. Also, several SIoTs and IoTs cooperate with each other through ‘‘bridge’’ objects, thus generating new architectures, referred to in the literature as Multiple IoT (MIoT) [21].